Data Fabric key to solving data challenges in a bank's digital transformation
Gartner predicts that a whopping 80 per cent of traditional banks will go out of business by 2030.
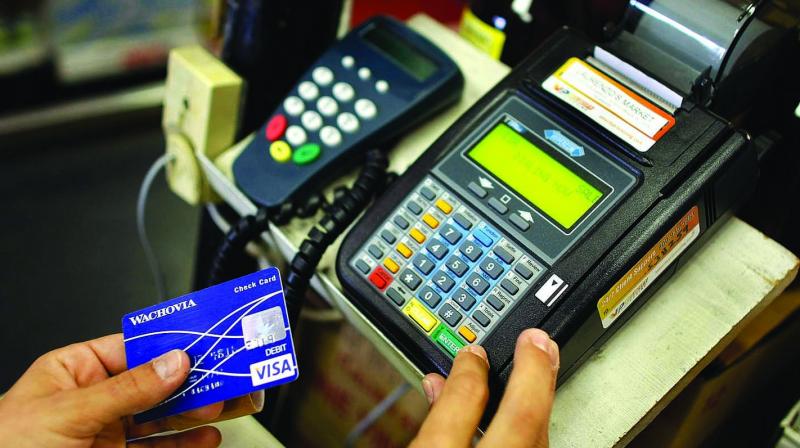
Gartner predicts that a whopping 80 per cent of traditional banks will go out of business by 2030. At the cusp of digital transformation, almost a third of financial services CIOs identified going digital as their top priority for 2019, up by 8 per cent from last year. Leaders face tremendous pressure in this competitive age to harness data at the right time, in the right quantity, and make the right insights to facilitate successful decision-making. While they don’t hesitate in making adequate investments, something hinders banks in leveraging this seemingly unlimited supply of data.
Embracing the paradigm design of Event-driven banking
Traditionally, banks have followed a request-driven model wherein a rigid architecture defines tasks. These systems are efficient in developing simple and set tasks, but fail to react to variable cases of the digital era. In the face of digital transformation, banks have significantly transformed their business models, culture, and operational models – taking them beyond the traditional banking systems. Faced by ever-changing regulations and a rapidly evolving technology landscape, banks are breaking down the legacy-era monoliths and adopting microservices as the core banking platform. In order to prevent loss of data, banking developers are moving to an event-driven architecture (EDA) system. But what is EDA?
Microservices perspective on design thinking: Challenges in the Banking Sector
Banks have been maintaining and operating monolith applications or legacy systems for a long time. Bringing in the microservices architecture is a challenge as it requires an investment of time, money, and a shift in mindset. Compared to the monolith architecture, a microservice architecture breaks down large software projects into inter-communicating modular units through APIs. This article explores how the future of design thinking would see its application across a complex environment of human behaviour and push boundaries of customer experience.
How Machine Learning eliminates “False Positives” in a bank’s AML Drive
Over the past several years, the instances of international money laundering have increased manifold, driving enforcement agencies in various countries to update their AML rules and regulations to curb illegal means of generating income. Globally, financial institutions are racing to deploy heightened screening systems and pushing their existing technologies to cope with the new rush. But enhanced identify verification systems are also a double-edged sword for Financial institutions and banks. As scrutiny becomes more strict, they also have to tread carefully so as not to cross the thin line of violating customer privacy. How does Machine Learning defeat the spectre of false positives in such a scenario?