Breast cancer can be diagnosed using machine learning
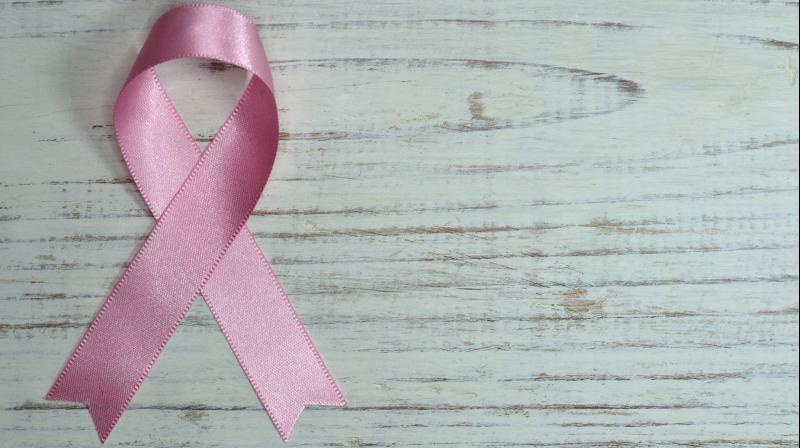
Breast ultrasound elastography is an emerging imaging technique used by doctors to help diagnose breast cancer, now researchers identified the critical role machine learning can play in making this technique more efficient and accurate in diagnosis.
Breast cancer is the leading cause of cancer-related death among women. It is also difficult to diagnose. Nearly one in 10 cancers are misdiagnosed as not cancerous, meaning that a patient can lose critical treatment time.
On the other hand, the more mammograms a woman has, the more likely it is she will see a false-positive result. After 10 years of annual mammograms, roughly two out of three patients who do not have cancer will be told that they do and be subjected to an invasive intervention, most likely a biopsy.
Using more precise information about the characteristics of a cancerous versus non-cancerous breast lesion, this methodology has demonstrated more accuracy compared to traditional modes of imaging.
In the case of breast ultrasound elastography, once an image of the affected area is taken, the image is analysed to determine displacements inside the tissue. Using this data and the physical laws of mechanics, the spatial distribution of mechanical properties like its stiffness is determined.
After this, one has to identify and quantify the appropriate features from the distribution, ultimately leading to a classification of the tumor as malignant or benign. The problem is the final two steps are computationally complex and inherently challenging.
In the research published in the journal of Computer Methods in Applied Mechanics and Engineering one of the researchers of the study, Assad Oberai sought to determine if they could skip the most complicated steps of this workflow entirely.
Cancerous breast tissue has two key properties: heterogeneity, which means some areas are soft and some are firm and non-linear elasticity, which means the fibres offer a lot of resistance when pulled instead of the initial give associated with benign tumors.
Knowing this, Oberai created physics-based models that showed varying levels of these key properties. He then used thousands of data inputs derived from these models in order to train the machine learning algorithm.
"If you had enough data available, you wouldn't. But in the case of medical imaging, you're lucky if you have 1,000 images. In situations like this where data is scarce, these kinds of techniques become important," said Oberai.
Through enough examples, the algorithm is able to glean different features inherent to a benign tumor versus a malignant tumor and make the correct determination.
Oberai and his team achieved nearly 100 per cent classification accuracy on other synthetic images. Once the algorithm was trained, they tested it on real-world images to determine how accurate it could be in providing a diagnosis, measuring these results against biopsy-confirmed diagnoses associated with these images.
"We had about an 80 per cent accuracy rate. Next, we continue to refine the algorithm by using more real-world images as inputs," Oberai said.